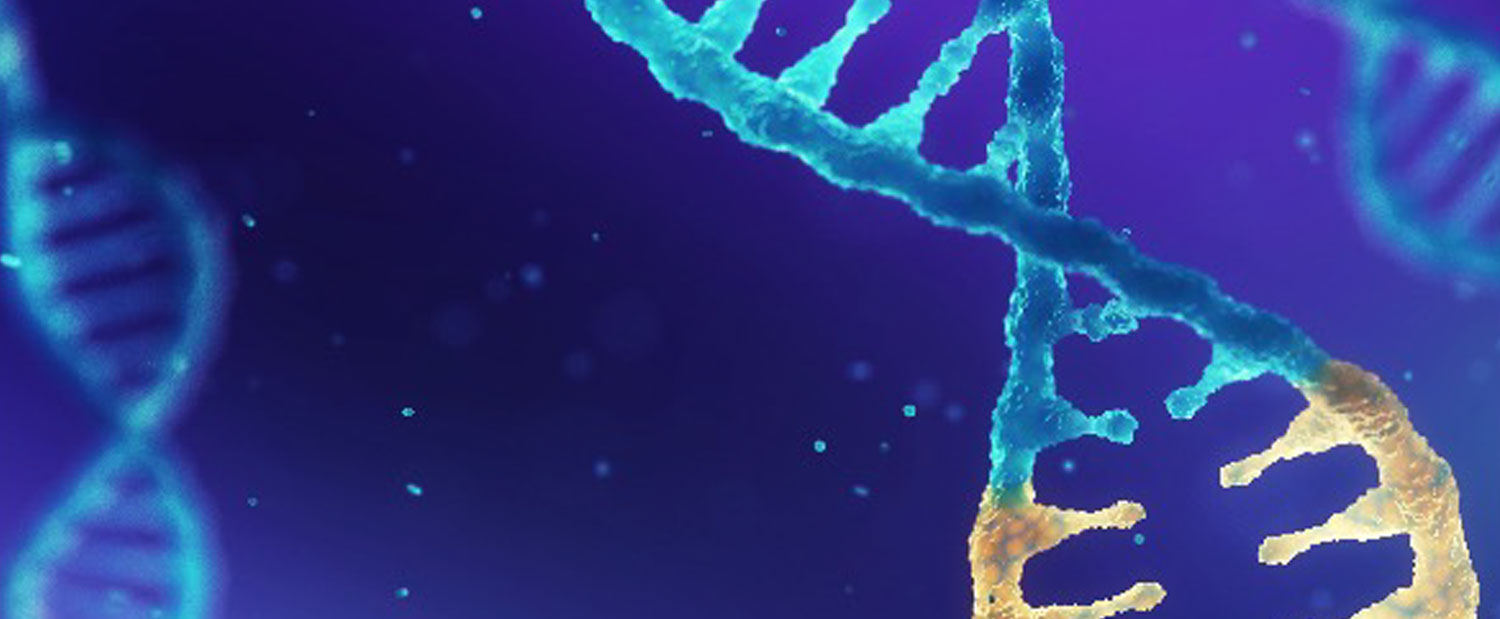
SPECIAL SERVICES FACILITY
In this issue of the newsletter, we will be highlighting the research being carried out by the Special Services Facility at DDI, led by Dr. Mohamed Abu-Farha.
RESEARCH UPDATE
Find out what is new within the Research Sector at DDI
SNP Genotyping Pipeline: A Discovery And Innovation Pipeline
Published on 19/10/2019
DDI is a pioneering center in genetic research regionally and internationally. Alongside whole genome and whole exome sequencing, an inexpensive technology that investigates genetic variation within the human genome is Single-nucleotide polymorphisms (SNPs) genotyping. SNPs are natural variants that occur within the human genome at a frequency that is above 1%. These variants can fall within the coding sequences of genes, non-coding regions, or regions between genes. These genetic variations have been associated with various diseases such as diabetes, obesity, cardiovascular disease and cancer. SNPs can cause disease through changing the coding amino acid modifying the protein structure and function or through modifying the regulatory binding elements in the promoter regions amongst other modalities. One of the best studies examples of SNPs involved in obesity is the fat mass and obesity-associated (FTO) gene which is a well-established obesity-susceptibility locus. Early genome-wide association studies (GWAS) associated FTO genetic variants with obesity traits in Europeans (Dina, Meyre et al. 2007, Frayling, Timpson et al. 2007); subsequently, many of these risk variants were replicated in Africans, South and East Asians and other populations. Furthermore, association of FTO variants with type 2 diabetes mellitus (T2DM), independent of body mass index (BMI), has been demonstrated in East and South Asians (Li, Kilpelainen et al. 2012) and Scandinavians (Hertel, Johansson et al. 2011).
The most notable genetic variants from FTO associated with obesity traits are a cluster of at least 20 common SNPs from its first intron (Loos and Yeo 2014). Despite the association of many FTO genetic variants with obesity traits in humans, elucidation of the mechanistic basis for these associations has been elusive. Obesity has reached epidemic proportions and understanding its genetic basis will help mitigate obesity-associated illness. In this context, there is an urgent need to decipher the causal mechanism for the role of FTO noncoding variants in the etiology of obesity and its related disorders (Wu and Arora 2016). As a result, DDI research sector has been interested in establishing a pipeline that can facilitate studying such variants within Kuwaiti population and enhance our understanding of the genetic causes of the high rates of obesity and diabetes in Kuwait. A discovery and innovation SNP genotyping pipeline have been already established and is currently being utilized by DDI researchers as we demonstrate in the publication example below. The various Components of the SNP genotyping system are shown in Figure 1 and are explained below:
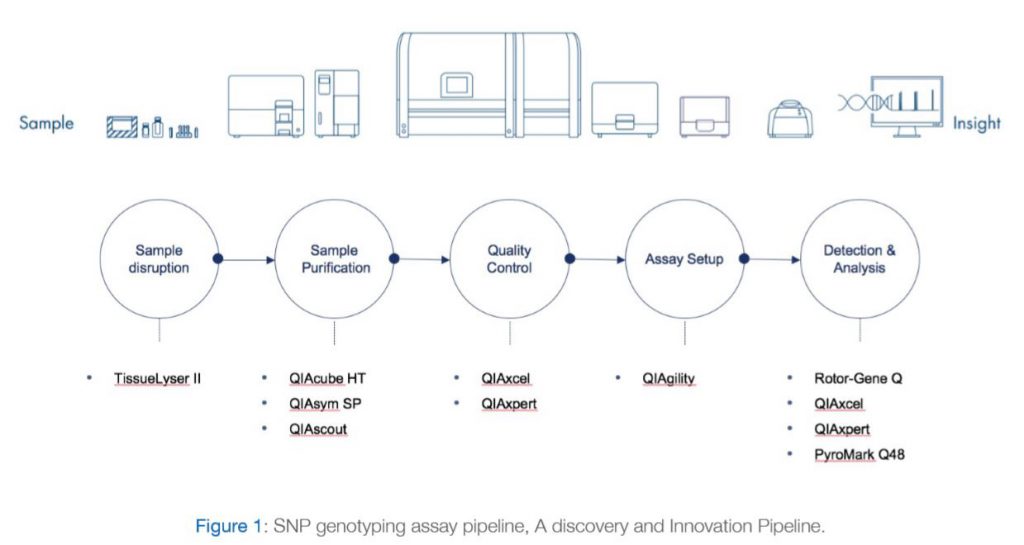
SAMPLE DISRUPTION
The TissueLyser II simultaneously disrupts multiple biological samples through high-speed shaking in plastic tubes with stainless steel, tungsten carbide, or glass beads. Using the appropriate adapter set, up to 48 or 192 samples can be processed at the same time. Alternatively, a grinding jar set can be used to process large samples. A range of beads, bead dispensers, and collection microtubes and caps are also available.
SAMPLE PURIFICATION
With a dedicated range of QIAsymphony Kits, the QIAsymphony SP enables sample preparation of DNA, RNA, and bacterial and viral nucleic acids from a wide range of starting materials. Figure 2 illustrates the workflow of the system.
Alternatively, QIAcube HT can be used with a variety of Purification Kits for quick and easy purification of DNA, RNA and miRNA from almost any type of sample – including cells, tissues, as well as from bacteria and viruses in animal samples.
QUALITY CONTROL
For effortless DNA and RNA analysis. The QIAxcel Advanced system fully automates sensitive, high-resolution capillary electrophoresis of up to 96 samples per run.
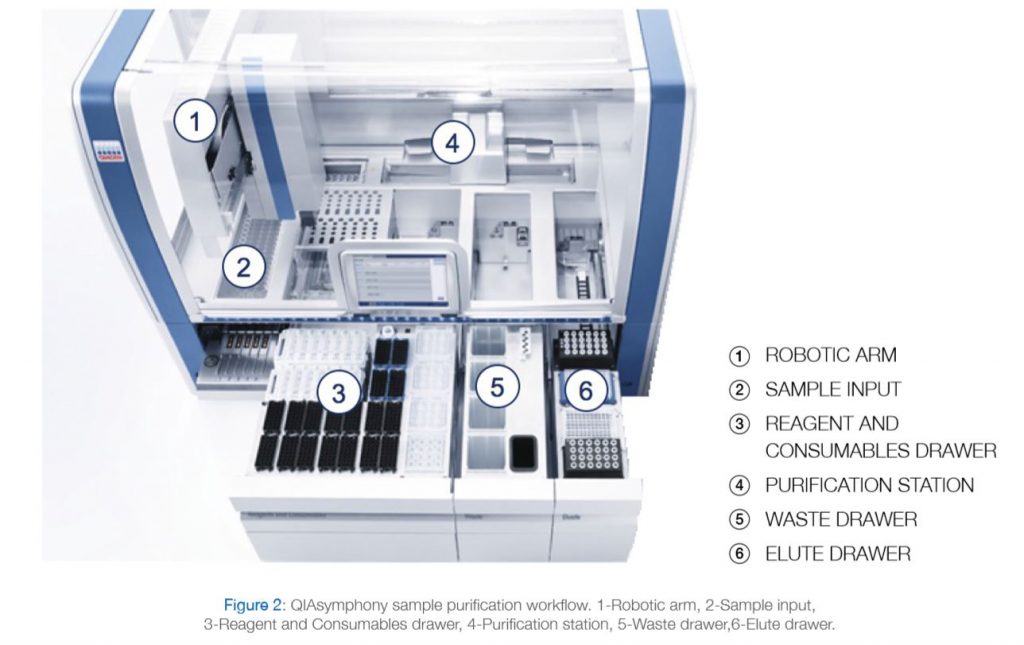
ASSAY SETUP
The QIAgility uses a single-channel pipet to automate PCR setup. Liquid-level sensing, facilitated by conductive filtered tips, enables high-precision, standardized pipetting. Automated PCR setup is rapid and reliable and eliminates manual pipetting steps that can be prone to human error. The QIAgility fully automates trusted QIAGEN PCR and RT-PCR, and real-time PCR and RT-PCR kits, and can set up multiple master mixes and can process multiple PCR setups in parallel. The advanced design of the QIAgility delivers improved process safety, giving you peace of mind. An in-built sensor ensures that the instrument pauses when the hood is opened during a run, protecting users from moving parts. An UV light ensures effective worktable decontamination and an HEPA filter provides positive clean air pressure throughout PCR setup.
DETECTION AND ANALYSIS
Rotor-Gene Q provides outstanding performance in real-time PCR as demonstrated below:
- No optical normalization and no reference dye (ROX) needed
- No optical calibration needed
- Lifetime guarantee on highly-stable LEDs; no expensive lamps or lasers to change,no gradual performance loss
- No block to clean
- No condensation or bubbles in reactions due to rotation
- Fewest moving parts and shortest optical path of all qPCR systems
- Self-configuring USB connection to computer
- Small, lightweight (14 kg), and robust. Simply place the instrument where you want and GO!
PyroMark Q48 Pyrosequencing machine with integrated template preparation for advanced methylation, mutation and SNP detection and quantification. Please refer to figure 3 for further explanations.
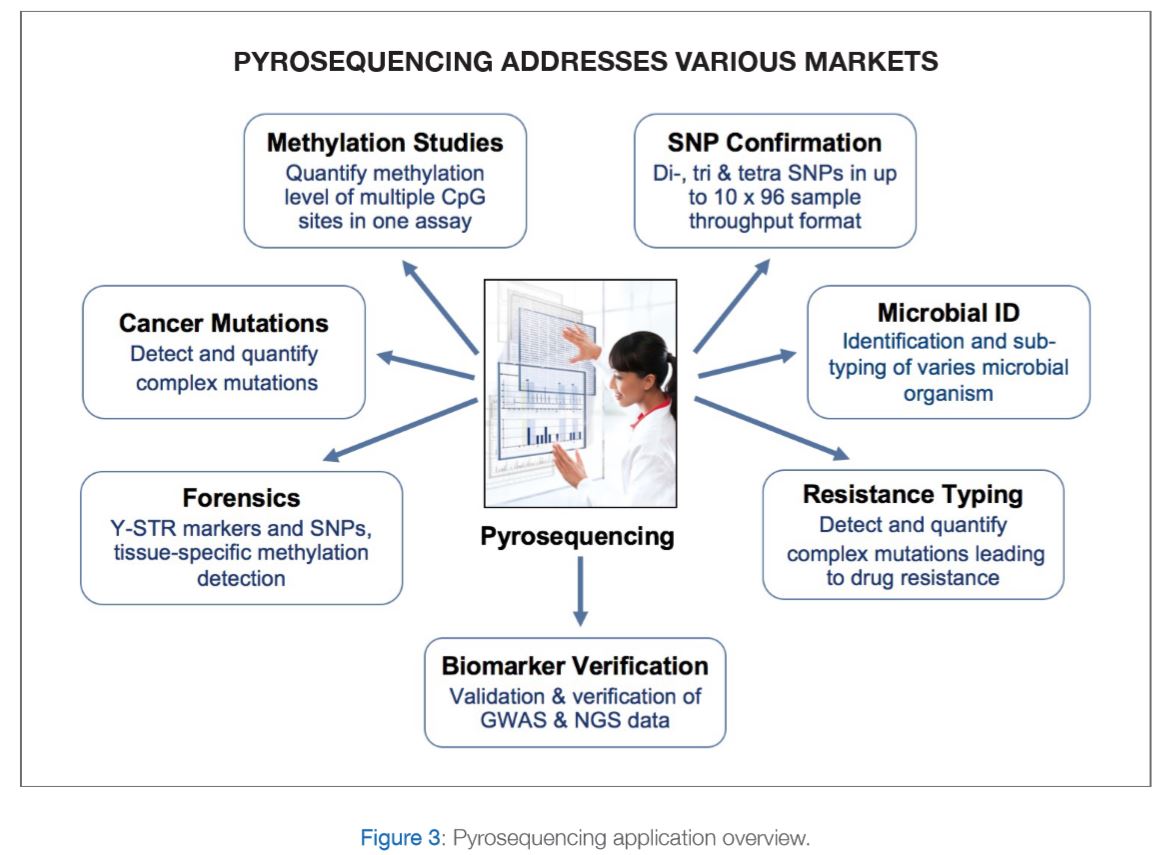
REFERENCES:
Dina, C., D. Meyre, S. Gallina, E. Durand, A. Korner, P. Jacobson, L. M. Carlsson, W. Kiess, V. Vatin, C. Lecoeur, J. Delplanque, E. Vaillant, F. Pattou, J. Ruiz, J. Weill, C. Levy-Marchal, F. Horber, N. Potoczna, S. Hercberg, C. Le Stunff, P. Bougneres, P. Kovacs, M. Marre, B. Balkau, S. Cauchi, J. C. Chevre and P. Froguel (2007). “Variation in FTO contributes to childhood obesity and severe adult obesity.” Nat Genet 39(6): 724-726.
Frayling, T. M., N. J. Timpson, M. N. Weedon, E. Zeggini, R. M. Freathy, C. M. Lindgren, J. R. Perry, K. S. Elliott, H. Lango, N. W. Rayner, B. Shields, L. W. Harries, J. C. Barrett, S. Ellard, C. J. Groves, B. Knight, A. M. Patch, A. R. Ness, S. Ebrahim, D. A. Lawlor, S. M. Ring, Y. Ben-Shlomo, M. R. Jarvelin, U. Sovio, A. J. Bennett, D. Melzer, L. Ferrucci, R. J. Loos, I. Barroso, N. J. Wareham, F. Karpe, K. R. Owen, L. R. Cardon, M. Walker, G. A. Hitman, C. N. Palmer, A. S. Doney, A. D. Morris, G. D. Smith, A. T. Hattersley and M. I. McCarthy (2007). “A common variant in the FTO gene is associated with body mass index and predisposes to childhood and adult obesity.” Science 316(5826): 889-894.
Hertel, J. K., S. Johansson, E. Sonestedt, A. Jonsson, R. T. Lie, C. G. Platou, P. M. Nilsson, G. Rukh, K. Midthjell, K. Hveem, O. Melander, L. Groop, V. Lyssenko, A. Molven, M. Orho-Melander and P. R. Njolstad (2011). “FTO, type 2 diabetes, and weight gain throughout adult life: a meta-analysis of 41,504 subjects from the Scandinavian HUNT, MDC, and MPP studies.” Diabetes 60(5): 1637-1644.
Li, H., T. O. Kilpelainen, C. Liu, J. Zhu, Y. Liu, C. Hu, Z. Yang, W. Zhang, W. Bao, S. Cha, Y. Wu, T. Yang, A. Sekine, B. Y. Choi, C. S. Yajnik, D. Zhou, F. Takeuchi, K. Yamamoto, J. C. Chan, K. R. Mani, L. F. Been, M. Imamura, E. Nakashima, N. Lee, T. Fujisawa, S. Karasawa, W. Wen, C. V. Joglekar, W. Lu, Y. Chang, Y. Xiang, Y. Gao, S. Liu, Y. Song, S. H. Kwak, H. D. Shin, K. S. Park, C. H. Fall, J. Y. Kim, P. C. Sham, K. S. Lam, W. Zheng, X. Shu, H. Deng, H. Ikegami, G. V. Krishnaveni, D. K. Sanghera, L. Chuang, L. Liu, R. Hu, Y. Kim, M. Daimon, K. Hotta, W. Jia, J. S. Kooner, J. C. Chambers, G. R. Chandak, R. C. Ma, S. Maeda, R. Dorajoo, M. Yokota, R. Takayanagi, N. Kato, X. Lin and R. J. Loos (2012). “Association of genetic variation in FTO with risk of obesity and type 2 diabetes with data from 96,551 East and South Asians.” Diabetologia 55(4): 981-995.
Loos, R. J. and G. S. Yeo (2014). “The bigger picture of FTO: the first GWAS-identified obesity gene.” Nat Rev Endocrinol 10(1): 51-61. Wu, C. and P. Arora (2016). “Noncoding Genome-Wide Association Studies Variant for Obesity: Inroads Into Mechanism: An Overview From the AHA’s Council on Functional Genomics and Translational Biology.” J Am Heart Assoc 5(7).